【2020 Application Example】 Small and Medium Enterprises AI Competency Evaluation System, Significantly Reducing the Cost of Competency Implementation for Businesses!
IBM's supercomputer Watson can predict when employees are likely to resign, with an accuracy rate of 95%, saving IBM up to $300 million a year in retaining employees. Moreover, through cloud computing services and modernization, IBM has streamlined 30% of personnel costs, allowing the remaining employees to earn higher salaries and engage in more valuable work.
So, in Taiwan, how can we ensure that 'employees who stay can receive higher salaries and perform more valuable work'? The key lies in the 'competence setting' for each position. According to the 'iCAP Competency Development Application Platform' established by the Ministry of Labor's Workforce Development Agency, every position has its main responsibilities, work tasks, behavioral indicators, work outputs, knowledge, skills, and attitudes. Only by establishing 'competency' for each position can enterprises effectively apply this in employee recruitment, education and training, and performance management. Without this, not knowing what employees should do is like groping in the dark, which can pose risks to business operations.
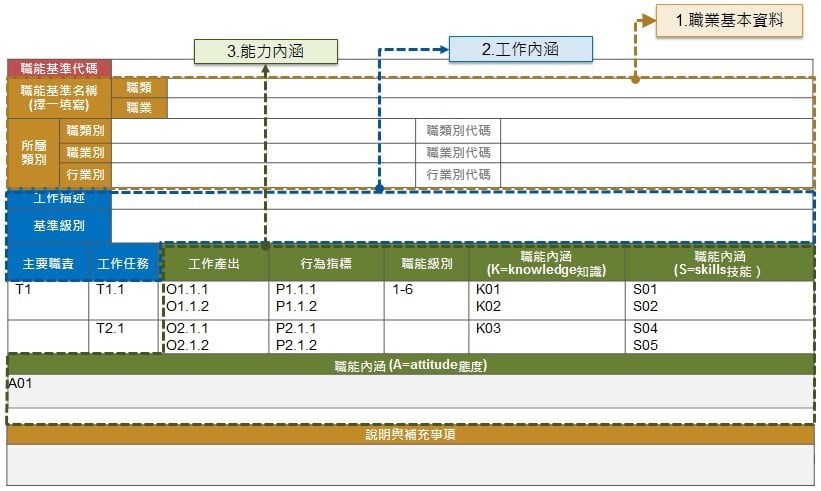
▲Competency Benchmark Example
Currently, on the 'iCAP Competency Development Application Platform', there are 872 established competency benchmarks, including 553 items completed by various ministries. This includes 253 items from the Ministry of Labor and 66 items from the Ministry of Education. If companies want to establish their own 'competency benchmarks', they need to search for reference materials on the 'iCAP Competency Development Application Platform'. Suppose a company wants to recruit 'sales' personnel but doesn't know what 'sales personnel' should do; they should first search for 'sales personnel' as shown in the figure below.
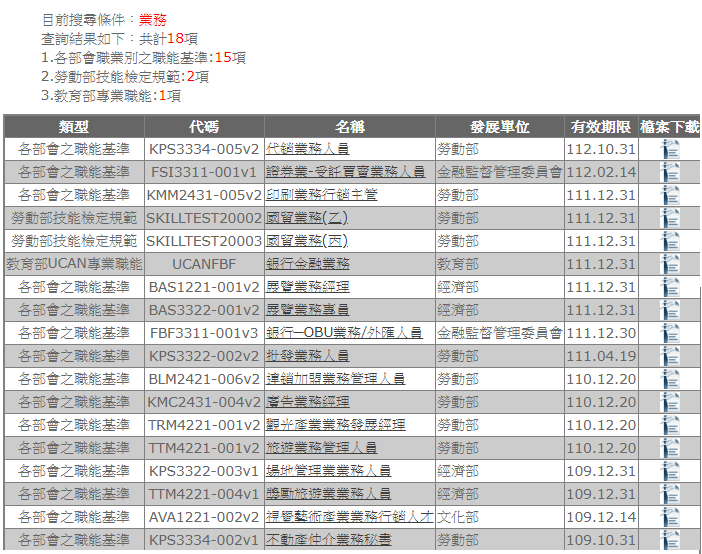
▲Searching for 'sales' on the 'iCAP Competency Development Application Platform'
You can find that there are 18 types of sales personnel. At this point, the company needs to go through each one, check, read, and organize into the 'competency benchmarks' they need; however, if we search what should be a common position in any company, 'general affairs', the result is unexpectedly zero items.

▲Searching for 'general affairs' on the 'iCAP Competency Development Application Platform'
As seen above, although the 'iCAP Competency Development Application Platform' established by the Ministry of Labor's Workforce Development Agency can solve some of the 'competency benchmarks' for positions, the division of labor within each company is different, and some positions might not be found on the 'iCAP Competency Development Application Platform'. Secondly, in small and medium enterprises, there are often 'multi-skilled workers', meaning many job responsibilities are on a single employee. For example, in small enterprises with less than 30 people, usually, accounting, general affairs, and HR are handled by the same person. If you want to establish competency benchmarks for this person, you have to search separately for 'accounting', 'general affairs', and 'HR', and then integrate these three types of job competencies, which is often time-consuming and ineffective.
This 'Small and Medium Enterprises AI Competency Evaluation System' aims to let 'people fully utilize their capabilities', by introducing AI to more accurately establish basic competency standards for employees, and to track their competency performance at any time.
Competency models are all generated and adjusted manually, which is time-consuming
A domestic exporter of screws, nuts, fasteners, etc., had all its competency models generated and adjusted manually. The execution process was time-consuming and insufficient to meet company needs due to personnel changes, such as: previously, Qiao Mai Enterprise had specialized 'production control personnel', but after this personnel resigned, this job had to be done by other employees, meaning other employees' competency models needed to be adjusted immediately. Or if the company needed to set up a development department due to future development, but previously no one had relevant experience, not only did they not know how to select from within, but also did not understand how to describe on a recruitment website to find the talent they really wanted.
Besides, the CEO of this company has always been troubled by internal performance management. Due to the lack of precise standards and systems to measure employee performance, the results of each performance assessment did not accurately reflect the true performance of the employees, forming assessment blind spots and unable to identify truly deserving employees. Thus, it is hoped that with the AI competency evaluation system, the necessary competencies for the development department can be immediately clarified, as well as how recruitment and performance appraisals should be conducted, so as to effectively solve the pain of unclear responsibilities and inaccurate assessments within the company. Thus, its benefits are significant!
AI Competency System Establishment X Deep Learning
This 4-month HR field competency system project has a clear execution direction, but the introduction of explanatory models such as Seq2Seq, Deep Keyphrase Generation, Tf-IDF keyword extraction algorithms, and PageRank are new attempts in the HR field. During the process, open-source big data architecture is used for natural language processing to complete Word2Vector and index, and inverted index to establish keyword weight and relevance. Due to the inability to process like image data with continuous numbers, it is necessary to simplify the feature values with related keywords such as skills, knowledge, and job categories. Basic steps are briefly described as follows:
1. Establish a Propagation model using Google's long-used LTR mixed Pointwise recommendation engine (2 months)
2. Establish a Back Propagation model (2 months), adjust the hyperparameters of the loss function
3. Adjust the hyperparameters of the CF model
4. Establish a human-machine collaboration mechanism to obtain more data to feed the Model 5. Repeat the above steps
During the development process of the competency model, Lianhe Trend Co., Ltd. and Weiguang International Information Co., Ltd. held multiple discussions, believing that there are interconnections between competencies. After establishing the knowledge graph, further upload the competency scale to the Neo4j graph database for processing complex relational data structures with excellent performance. Currently, 500 competency scales have been uploaded to the Neo4j relationship analysis platform.
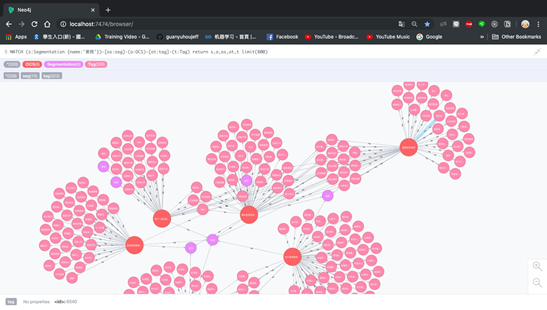
▲Using python for wor2vector natural language analysis
In addition to describing a position with a tensor after word2vector, finding out the appearance of this position's knowledge graph, according to this knowledge graph, one can understand the relevance between different positions and the similarity performance of their dimensions. Finally, this knowledge graph is used to establish the company's 'competency model' and train it with deep learning.
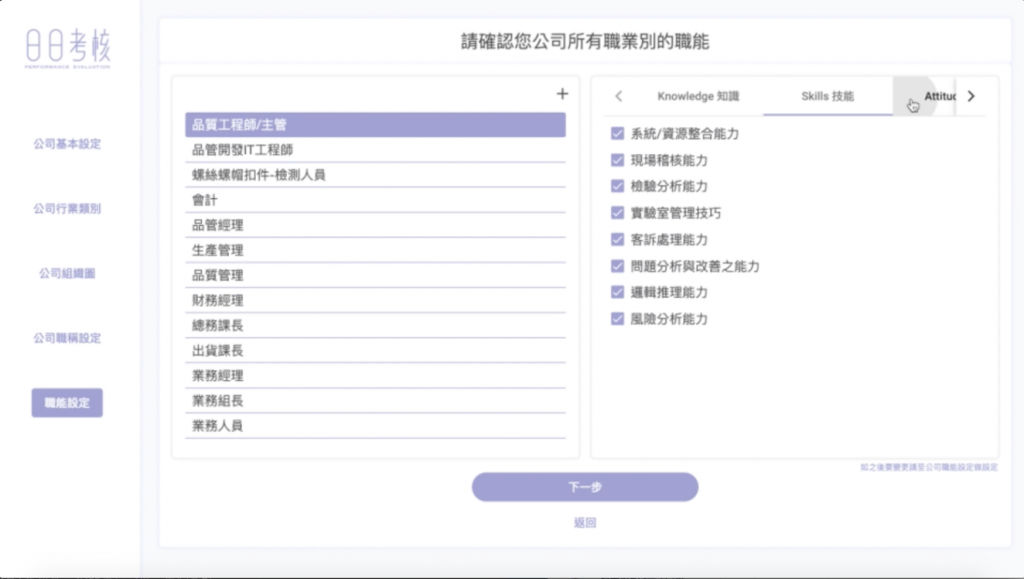
▲AI Competency Evaluation System Interface
In the future, in addition to establishing their own competency models, companies can also be opened to end-users. Individuals can analyze their own competency performance to understand their possibilities for job change and their market value, as well as identify skills needing enhancement. If companies respond to this knowledge graph, they can develop cross-industry products in the future.
1. Short-term: Analyze the competency scales (iCAP, iPAS) published by the government with natural language and keyword models, and cooperate with unsupervised learning to establish 'Native Competency Base Unit Models'.
2. Medium-term: Tailor-made exclusive competency models for enterprises. Based on the existing 'Native Competency Base Unit Models', experts use supervised learning to train the individual company's 'Distributed Derivative Competency Models'.
3. Long-term: Establish 'Reinforcement Learning' models, incorporating employee career cognition and planning.
Competency model recommendations, comparable to professional human resource consultants
Through the dynamic learning of the competency knowledge graph through unsupervised learning, individual companies' competency models are quickly established. Internal human resources personnel or external professional HR consultants can then use the generated competency models to assess and apply aspects of talent recruitment, competency inventory, performance management, and education and training. The system will automatically suggest competencies to be strengthened according to the company's existing job structure, including related knowledge, skills, and attitudes. Through the continuous introduction and training of data, the system learns the employer's actual view of the model for that profession and feeds back to the cloud competency scale, completing the dynamic learning of the knowledge graph through transfer learning. In the future, it can be comparable to professional HR consultants, thereby rapidly assisting many cross-disciplinary or technologically diverse companies in training employee competencies.
「Translated content is generated by ChatGPT and is for reference only. Translation date:2024-05-19」