【2021 Application Example】 Maintaining the reputation of the “Kingdom of Yachts” - Kha Shing Enterprise introduces the first domestic FRP ultrasonic smart inspection of composite materials

The Kaohsiung-based Kha Shing Enterprise Co., Ltd. was established over 40 years ago, and is Taiwan's largest customized yacht company with customers all over America, Europe, Asia, and Australia, earning Taiwan the reputation of the "Kingdom of Yachts." Current FRP hull inspection still relies on traditional methods, such as visual inspection and knocking sounds, which is time-consuming and labor-intensive. Kha Shing has applied PAUT array ultrasonic inspection to hull FRP composite materials for the first time, and combined it with AI to interpret ultrasound images, develop complete intelligent solutions, and create emerging markets for inspection companies.
Kha Shing Enterprise Co., Ltd. was formerly Kha Shing Wood Industry Co., Ltd., and was a factory specializing in wood import in Kaohsiung Linhai Industrial Park when it was first established. It began to design, manufacture, and sell yachts in 1977. After the second-generation successor of the company, President Kung Chun-Hao entered the company, he made a breakthrough in the previous manufacturing model that relied mainly on the skills of master craftsmen, introduced digital manufacturing to accelerate shipbuilding, and began to make larger yachts, ranking in the top 20 manufacturers worldwide among manufacturers of large yachts over 24 feet. It also set a record of delivering 94 yachts within one year, earning Taiwan the reputation of "Kingdom of Yachts."
Defect detection ensures yacht quality, using AI to replace humans to achieve higher efficiency
Defect detection is very important to ensuring yacht quality. At present, the yacht industry still uses very traditional defect detection methods. The hull structure is usually made by hand lay-up or the vacuum infusion process, using visual inspection or knocking and the frequency of the sound to determine defects. It requires time-consuming manual inspection. If there are any defects, they must be reworked and repaired, and a gel coat subsequently sprayed. The hull must be constructed in sections to facilitate inspection. For large yachts over 24 meters long, construction in sections is very time-consuming and labor-intensive.
To shorten the time of the yacht manufacturing process, Kha Shing Enterprise will first carry out the gel coating process for the hull, and then perform the hand lay-on process. The hull manufacturing process has two types of composite material test specimen structures. In terms of 54-foot yacht hulls, the hull contains gel coat, core material, fiber and resin, and the total thickness is about 3.2cm±0.1cm, which is twice the total thickness of FRP hull without core material of about 1.6cm±0.1cm. Defects such as incomplete impregnation of glass fiber or residual air bubbles between glass fiber and resin occasionally occur during the manufacturing process. The types of defects include insufficient resin, voids, and delamination. Once defects occur, the supply of hull materials will be insufficient and yacht delivery will be delayed.
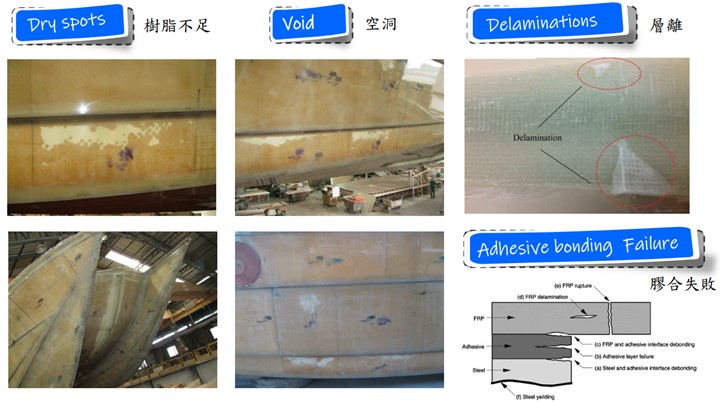
▲Schematic diagram of types of FRP hull.
In order to solve this problem, Kha Shing Enterprise has engaged in technical cooperated with the metal materials industry and the AI technology industry, combining the ultrasonic inspection expertise of the metal materials industry with AI technologies developed by the AI technology industry in recent years to help solve issues of Kha Shing Enterprise with defect detection. The method uses PAUT on the composite material structure of yachts, conducts FRP ultrasonic evaluation to determine the thickness of the yacht hull and material properties, and evaluates the ultrasonic probe frequency applicable to the hull structure based on professional ultrasonic experience. After testing, a frequency of 5MHz and a probe width of 45mm can successfully find the location and size of defects in the simulated defect test specimen.
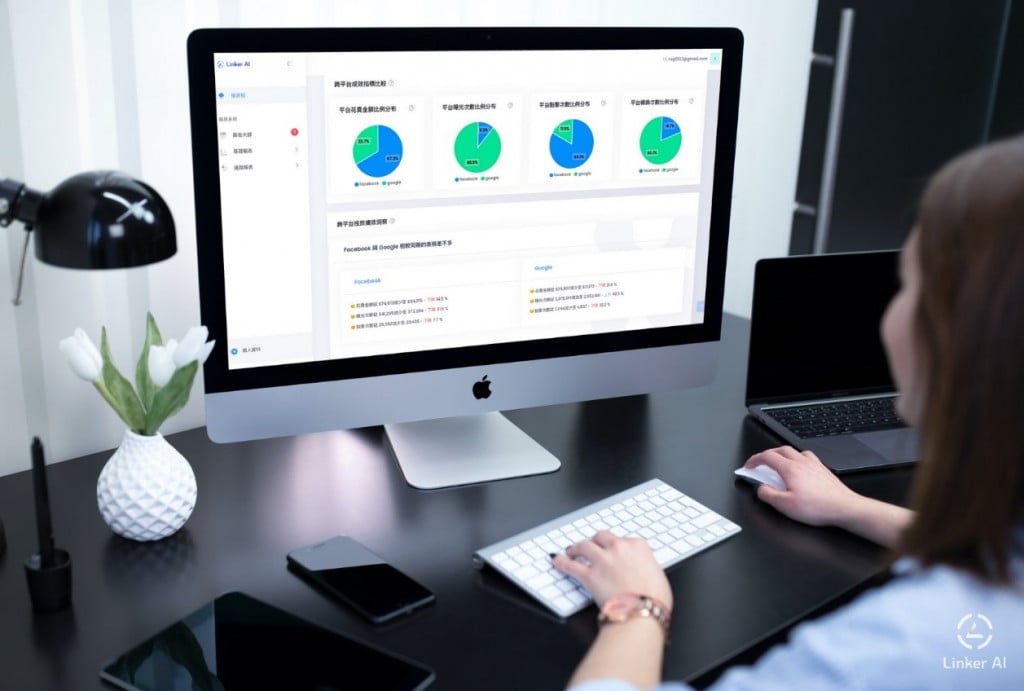
▲The three parties jointly found defect detection solutions from array ultrasonic evaluation, AI technology model development, and actual application in yachts.
The image inspected is an ultrasound image. The image displays different colors based on the ultrasonic feedback signal. An AI model that automatically identifies defective parts is established through the YOLO algorithm. If the amount of abnormal data collected is insufficient for training, the CNN-based Autoencoder algorithm is used to collect normal image data for training and construct an AI model for abnormality detection. The object detection YOLO model is trained by inputting image data marked as having defects, while the abnormality detection model is trained by inputting image data without defects.
▲Simulated defective specimen corresponding to PAUT results
Defect detection by and AI system can shorten the construction period by 1.5 months and speed up determination by 50%
After the development of this AI system is completed, it will be validated on actual 54-foot yachts of Kha Shing Enterprise, and can effectively resolve issues with defects. The application of AI technology in ultrasonic inspection for intelligent determination is expected to accelerate determination by approximately 50%, and will also shortens the construction period by 1.5 months, effectively improving the speed and quality of the yacht manufacturing process.
As Taiwan develops larger and more refined yachts, it will create opportunities for industry optimization and transformation, as well as opportunities for the development of key technologies. The application of an AI ultrasonic inspection solution for composite materials is the first of its kind in the yacht industry, and is expected to attract more yacht manufacturers with inspection needs. The AI ultrasonic inspection solution for composite materials has three major competitive advantages:
1. Professional inspection experience and digital database to facilitate process management and analysis.
2. Automatic AI determination and identification quickly identifies defects and provides immediate feedback to process engineers.
3. High-efficiency process inspection provides defect repair recommendations, reduces damage rate, and improves the strength and quality of composite materials.
The application of AI technology can optimize the yacht manufacturing process, reduce manual inspection, create added value through the application of AI in Taiwan’s yacht industry, increase international purchase orders, and allow Taiwan yachts to continue to enjoy a good reputation in the world. Furthermore, this business model has also spread to fields of application related to composite materials, increasing cross-sector market usage. It is estimated to contribute approximately NT$1.4 to NT$2 billion in economic benefits to Taiwan's equipment maintenance and non-destructive testing market.