【2020 Application Example】 Textile Industry Challenges Fast Fashion, AI Inventory Forecast Reduces Error Rate by 35%
Fast fashion in clothing, small quantities, diverse styles, short delivery times
The textile industry faces the impact of the fast fashion trend among clothing brands, affecting the entire supply chain. Global brand channels are promoting zero inventory, short delivery periods, and small-scale customization. Balancing production time, quality, and cost is challenging. Often, there is a discrepancy between ODM predictions and actual demands from brand owners, causing issues in material management and excessive inventory costs.
Due to inaccurate demand forecasts from customers, it often leads to difficulties in material preparation. Excessive materials can increase leftover stock, while insufficient materials may delay delivery. This project aims to establish an AI-based material demand forecast model specifically for major domestic manufacturers.
AI calculates sales trends to further predict demand
The advisory team collaborates with Shentong Information Technology to mainly use the LSTM algorithm for the AI foundation. The goal is to predict the next sales cycle based on past sales records, utilizing simple regression to complex 'Time Series Analysis' in statistics. Usually, a period's sales volume closely relates to the previous period's, unless there is a major event, in which case it would typically follow a pattern.
There are various patterns of sales volume forecasts, including revenue, profit, customer counts, park visits, sales number/amount, etc. This will take the example of a factory's monthly shipment batches, using the LSTM model to predict the next month's shipment batches.
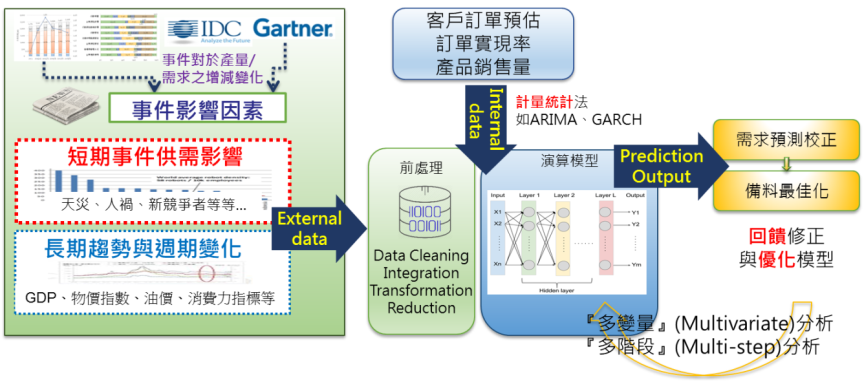
▲Material Demand Analysis Execution Framework
This project plans to establish a customer-specific material demand AI prediction model. During the planning phase, three different machine learning algorithms were used to prototype the AI model:
Logistic Regression Algorithm
Gradient Boosting Algorithm
Deep Learning Algorithm
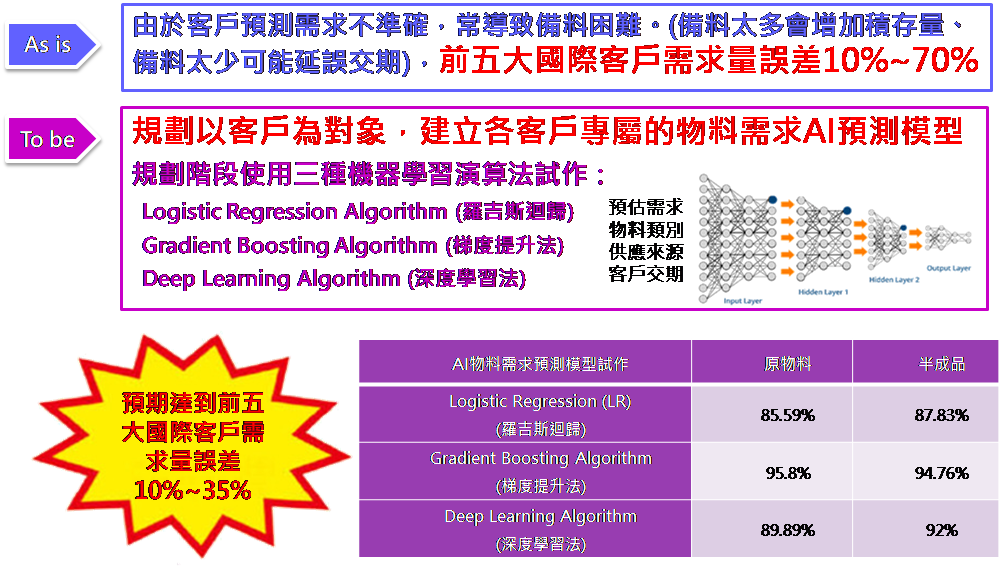
▲Material Demand AI Prediction Model Planning
Demand forecast error reduced from a maximum of 70% to 35%, significantly reducing inventory volumes
This project estimates customer demands, required material types, supply sources, and customer delivery dates using machine learning to establish a primary material procurement prediction system. It reduces the prediction error of demand from the top five international customers from a high of 70% to 35%, significantly lessening the amount of inventory needed.
「Translated content is generated by ChatGPT and is for reference only. Translation date:2024-05-19」