【2020 Application Example】 AI Bread Recognition System, machine scans, and the price is instantly calculated for you!
A brilliant idea transforming AI facial recognition technology
As artificial intelligence develops, more and more industries are embracing AI technology, even subtly entering into people's lives. As most bakeries sell freshly made bread and pastries, which typically do not have barcodes, they rely on cashiers to visually identify each item and enter the type and price of the bread. Thus, inspired by AI facial recognition technology, if such artificial intelligence could identify hundreds of types of bread, it could enhance checkout efficiency...
Diverse handmade breads delight customers but challenge clerks!
A local bakery has over 100 types of bread, regularly updating or adding new products, offering customers a variety of choices; this poses a challenge for cashiers.
It takes two months to train a cashier, but even after they start, there's still a 5 to 10% error rate due to bread recognition mistakes each month, especially during peak checkout times after work, causing bottlenecks and further errors due to the stress on cashiers. The difficulty in training cashiers and the lack of precision in the checkout process have long troubled businesses...
When baking meets artificial intelligence, it sparks a marvelous retail experience!
In typical bakeries, bread is sold 'naked' immediately after baking and then 'packaged' when it cools to room temperature. Both methods require cashiers to recognize and remember the prices and undergo two months of training before they can work the cash register. Even then, there is still a 5 to 10% error rate each month. My Dee Bakery, with its extensive range of over 100 bread types, poses a significant challenge for cashiers!
Due to Yun Kui Technology Co., Ltd.'s expertise in developing iPad POS systems, which are designed to be simple, convenient, and easy to use, they allow businesses to check out efficiently and accurately. Therefore, integrating the existing POS system with AI image recognition capabilities enables businesses to carry out transactions more efficiently and precisely.
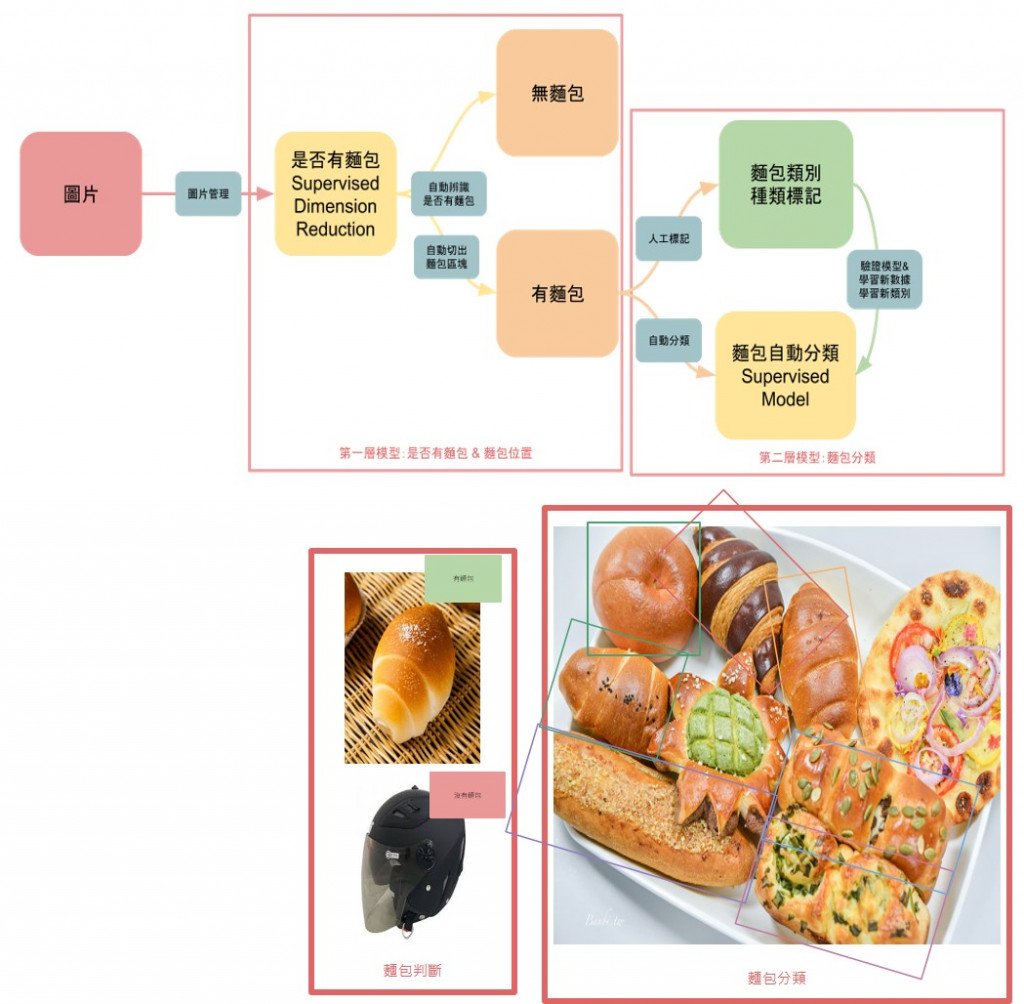
▲AI bread recognition model operational schematic (Image provided by Yun Kui Technology)
The execution can be simplified into eight steps, which include:
1. Data collection: Take bread image data at bakeries.
2. Image annotation: The image data is handed over to Mu Kesi Co., Ltd. for manual annotation.
3. AI modeling and training: Managed by Mu Kesi, who adjusts AI models and training.
4. iPad POS adjustment: Simultaneous adjustments of the UI interface on the POS side and backend integration with the AI model.
5. Start testing: Once Mu Kesi reaches over 95% recognition accuracy with current data, formal integration testing begins.
6. Real scene testing: Move to the bakery to gather data and verify the correct recognition rates.
7. Planning real scene application accessories: When recognition accuracy exceeds 98%, design accessories for on-site checkout, such as remote cameras and projection light sources.
8. Official Application: Integration with electronic receipts goes live.
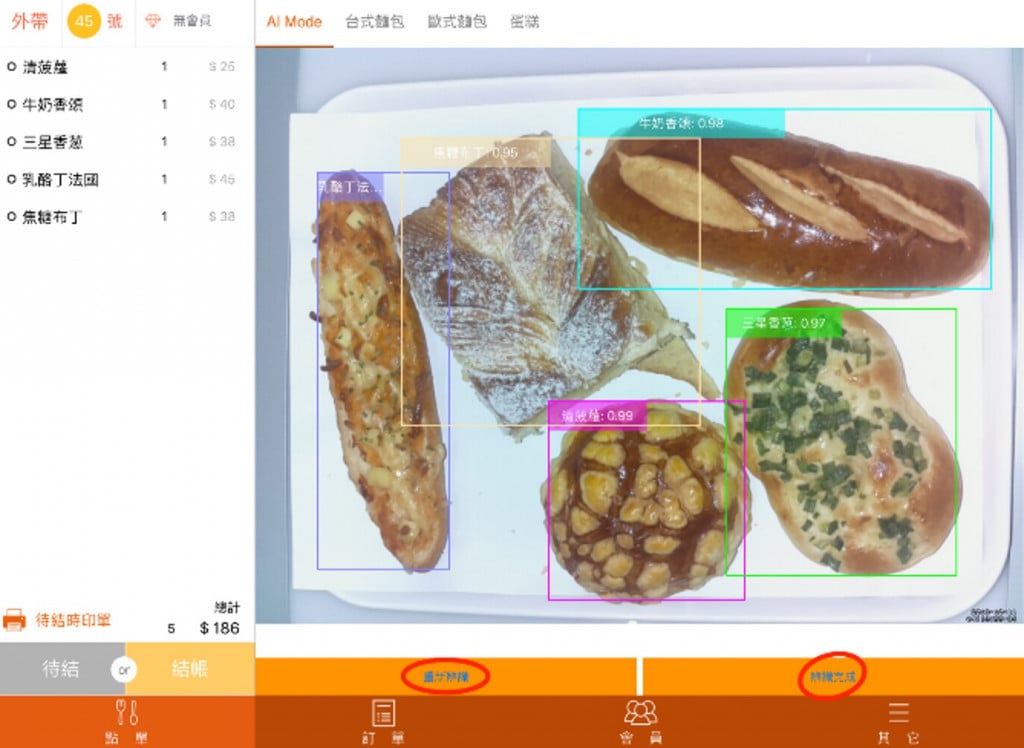
▲POS machine AI bread recognition checkout process: Start recognition - Recognition complete - Checkout - Confirm checkout, takes only 3 seconds (Image provided by Yun Kui Technology)
AI bread recognition system, making multitasking easy!
After adding AI capabilities, not only can it save upfront training time and costs for bakery cashiers and reduce costs from recognition errors, but it can also speed up the checkout process and efficiency, increasing customer satisfaction. This can later be promoted to various retail industries, expanding the new map of smart retail.
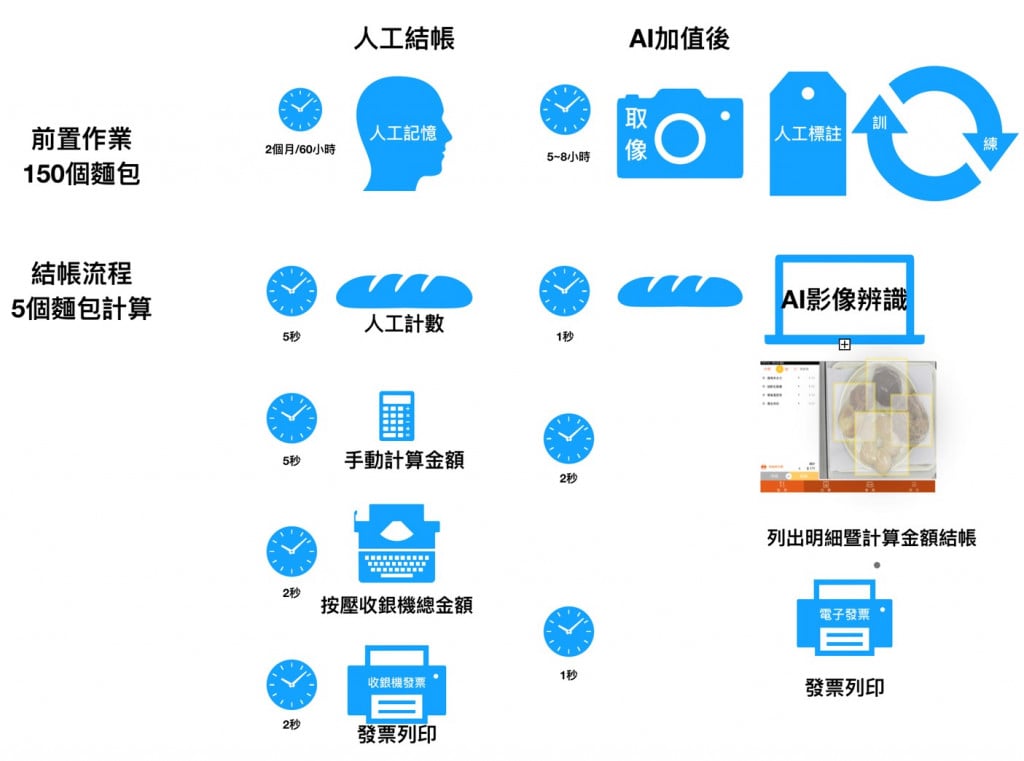
▲Before and after comparison chart of the bread checkout process with AI valuation (Image provided by Yun Kui Technology)
「Translated content is generated by ChatGPT and is for reference only. Translation date:2024-05-19」