【2021 Application Example】 Optical industry AOI imports AI Great Leap Forward to completely solve the pain points of lens defect detection

The stay-at-home economy such as smartphones and remote working is booming, and the information and communication industry is booming, driving the optical industry to flourish. However, the defect detection of optical lenses is mostly carried out by human eyes, which is not only time-consuming and labor-intensive, but also limited by the fact that human eyes are prone to fatigue. The misjudgment rate is also a lingering pain point for the optical industry. Benefiting from the evolution of AI technology, Shangyang Optics introduced diffraction optical technology for shooting, used the images captured by the system as the data source, introduced AI model training, and integrated the camera system and image recognition into a production line workstation, greatly improving defect identification The rate is as high as over 90%.
Taiwan’s optical production value accounts for 10% of the world’s, and the application range of precision optics is expanding day by day
The optical industry is a mainstream product in consumer electronics. Even though Taiwan was affected by the Sino-US trade dispute in 2019, the output value of optoelectronics still reached US$46.3 billion, accounting for 10% of the world's total. Among them, the "precision optics" segment accounts for NT$87 billion (approximately US$2.9 billion) in output value. In view of the increase in the number of smartphone lenses, precision optics still maintains a sustained growth of 4% compared to the decline in other fields.
Since Sharp launched the world's first camera phone equipped with a rear 110,000-pixel lens in 2000, end consumers' requirements for smartphone camera performance have continued to increase, and with the wave of 5G high-speed Internet The advent of the technology has led to the activation of application markets such as augmented reality (AR) or virtual reality (VR). The innovation and application of its technology have added a lot of momentum to the optical industry, and the application fields have extended from smartphones to popularization. to the mass consumer markets such as automobiles and home entertainment.
Optical lenses are inseparable from the economic development of "precision optics". As semiconductor technology continues to mature and network speeds continue to increase, optical lenses are used not only in smartphones, tablets, traditional cameras, projectors, In the field of people's livelihood vehicles, the demand for engineering visual inspection and security applications in high-precision manufacturing processes continues to grow rapidly.
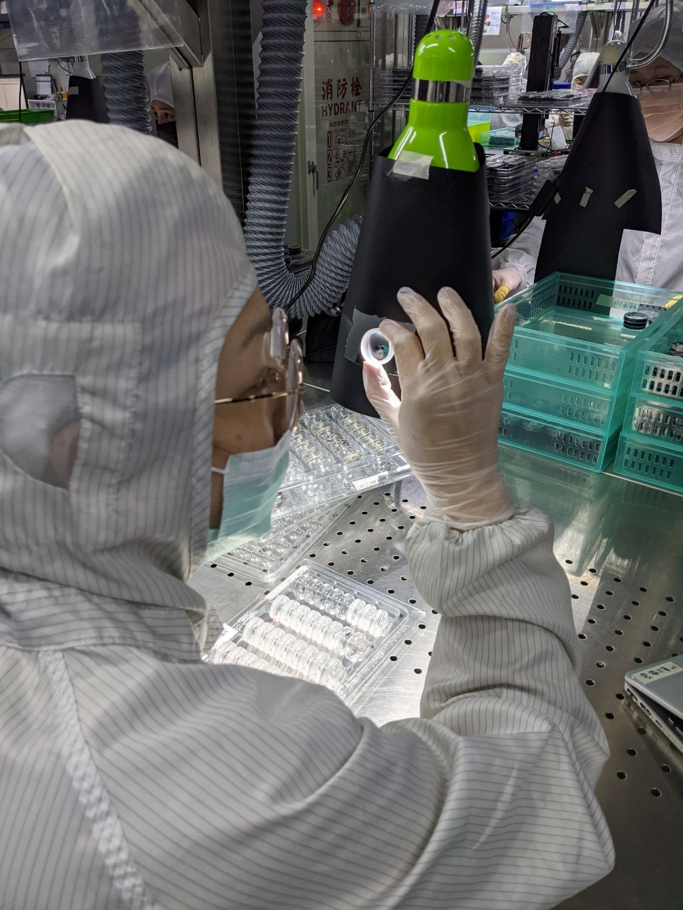
▲ Optical lens defect detection is mostly done manually.
"Optical lenses" are essential components of the overall optical-mechanical system. The lens finish inspection after incoming materials and before shipment not only affects the overall production line efficiency development, but also has an impact on the quality commitment of end customers that cannot be underestimated. For a long time, the optical industry has mostly used human eye detection for defect inspection. As production volume continues to increase, not only labor costs continue to rise. As inspectors age, their eyesight gradually declines, and the misjudgment rate increases every year. In addition, manpower recruitment has been difficult in recent years. Even if they are lucky enough to be recruited, it is not easy to develop the inspection technology, and the training time is lengthy, making it impossible to respond to the production line manpower needs in a timely manner.
Introducing diffraction optical technology and AI training model to improve defect recognition rate to more than 90%
The current market is flooded with a large number of automated optical inspection systems, and there are many substantial cases of lens defects. However, after years of market exploration and evaluation by Shangyang Optics, this system still cannot solve the current manual inspection problem. The main reason is that the appearance of the optical lens is curved and transparent, and it is not easy to photograph various defects, and once the defects are around There is interference from other stray lights, making judgment more difficult. Moreover, different types of lenses need to be individually rotated and lit and adjusted according to the defect status before entering the judgment stage. The labor consumption ratio is still high, which is not in line with the efficiency and cost.
Through this, through the matchmaking of the AI project execution team of the Industrial Bureau of the Ministry of Economic Affairs, Xiaoma Optics assisted Shangyang Optoelectronics in establishing an effective defect photography system. Pony Optics provides guidance on precision diffraction optics. Based on the characteristics of "light" fluctuations, lens defects can be obtained through a unified lens shooting method. Current photography systems on the market mostly use geometric optics. Geometric optics uses linear light and is not easy to capture defects such as missing coatings, tiny scratches, and liquid dirt. The cooperation plan introduces diffraction optical technology for shooting. Through precise imaging from all angles, it can achieve higher contrast and better noise reduction than ordinary geometric optical elements, so as to obtain the necessary defective images.
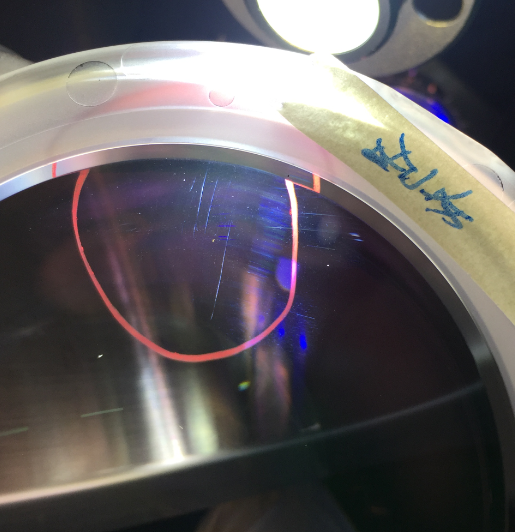
▲Schematic diagram of optical lens scratches and defects.
In order to improve the more detailed defect detection and recognition rate in this case, Shangyang Optics used the image captured by the system as the data source, imported AI model training, and integrated the camera system and image recognition into a production line workstation, which not only improved the defect recognition rate Reaching more than 90%, it is more conducive to the subsequent development of automated production lines.
The AI model training for this cooperation project is provided by Yirui Technology. Currently, most manufacturers have introduced AOI systems for production line defect inspection. Most of them use OCR (optical character recognition), which refers to the analysis and recognition processing of image files of text data. , the process of obtaining text and layout information) technology needs to be 100% accurate, and there is no room for error, resulting in accidental killings often occurring.
▲After adding the AI training model, the optical lens defect recognition rate is greatly improved.
AI+AOI solves the two major pain points of insufficient manpower and high misjudgment rate
This time, Yirui Technology and Xiaoma Optics cooperated to install Yirui's AI system in the optical inspection instruments developed by Xiaoma Optics, adding AI algorithms to the optical detection of defects, and training based on the data and needs provided by customers. AI model identification can greatly improve the accuracy of identification of defects, improve yield rate, and increase production line efficiency. Through the tripartite cooperation between Shangyang Optics, Xiaoma Optics and Yirui Technology, the optical industry AOI is introduced into AI, hoping to completely solve the pain points of industrial lens defect detection.
Since setting up the production line in 2019, Shangyang Optics hopes to introduce a smart production model. In view of the continuous growth of the company's operations and the continuous improvement of production volume, through the introduction and expansion of this achievement, the demand for manpower will be significantly reduced, and the impact of production scheduling can be reduced due to the high accuracy of the discrimination rate index, thereby improving production efficiency.
Shangyang Optics stated that as the development results are implemented, it will lead the technology to be promoted to upstream and downstream players in the optical industry, such as upstream optical lens raw material suppliers to downstream finished product applications, including immersive gaming equipment and related curved glass products , people's livelihood vehicle and security camera devices, etc.
「Translated content is generated by ChatGPT and is for reference only. Translation date:2024-05-19」