【2020 Application Example】 At-Home Laundry Smart Service System, through AI membership management, creating an intelligent laundry industry
Where to find a convenient and useful laundry service provider?
What to do when you want to send clothes for dry cleaning but can't reach them by phone to confirm if they are open today? Is the dry cleaning shop's APP space-consuming and not user-friendly? What if there are issues with the clothes after cleaning and there is no customer service system to handle complaints promptly?
According to statistics from the Directorate-General of Budget, Accounting and Statistics, the number of laundry businesses in Taiwan surpassed 6,000 in August 2019, making it a major challenge to stand out among many laundry providers.
Complaint Management, Dangerous Edge
A domestic dry-cleaning brand chain store launched a laundry app in mid-2015, featuring 'At-Home Laundry Collection and Delivery'. The app now has 20,000 downloads, approximately 6,000 members, and is actually used by about 300 people each month. Despite such convenient service, it has received many negative reviews from consumers, causing difficulties in expanding operations. The problems and improvement needs it faces are as follows:
1. Lack of incentives for consumers to download the app and the high costs:
Consumers need to download the app to use the service, and 'how to entice consumers to download' is the biggest challenge for the app service. The logistic costs are much higher than competitors due to the affordable, high-quality ideology with home collection and delivery service, and the costs of marketing the app make it difficult to achieve sustainable operation.
2. Staff shortages leading to customer service issues:
The original customer service method of the app was primarily by email. Due to insufficient staff, it was not possible to service by phone, thus delays in response and often overlooks of consumer issues occurred, leading to customer dissatisfaction.
Most customer complaints occur after the consumer receives the clothes and finds issues like missing items, damages, or color differences after washing. Upon receiving the complaint, customer service first requests photos of the laundry bag from the factory and then asks the consumer to provide photos of the received items for comparison. If it is concluded that the issue was not due to factory negligence, the factory-provided photos are sent to the consumer to clarify the matter. This customer service process requires a lot of manpower and time, seriously lacking in service efficiency!
Perfect AI Customer Service Experience
Siyan Technology Co., Ltd. and the AI team Chester International Ltd. collaborated to create the 'Smart Online Reservation Service System' through data analysis and intelligent customer service, facilitating online appointment and home collection of laundry services and building a 24-hour reservation and customer response service.
The intelligent customer service adopts the latest artificial intelligence deep learning, automatically records each Q&A session, possesses error correction capabilities, and introduces new services like customer service forms, push notifications, customer service robots, and LINE human customer support, greatly improving the convenience of customer contact and confirmation, significantly shortening customer service response times, and also providing more immediate services. Through data analysis, an automated AI membership management strategy is created, effectively increasing consumer repurchase rates and satisfaction.
▲1-on-1 LINE Human Customer Service
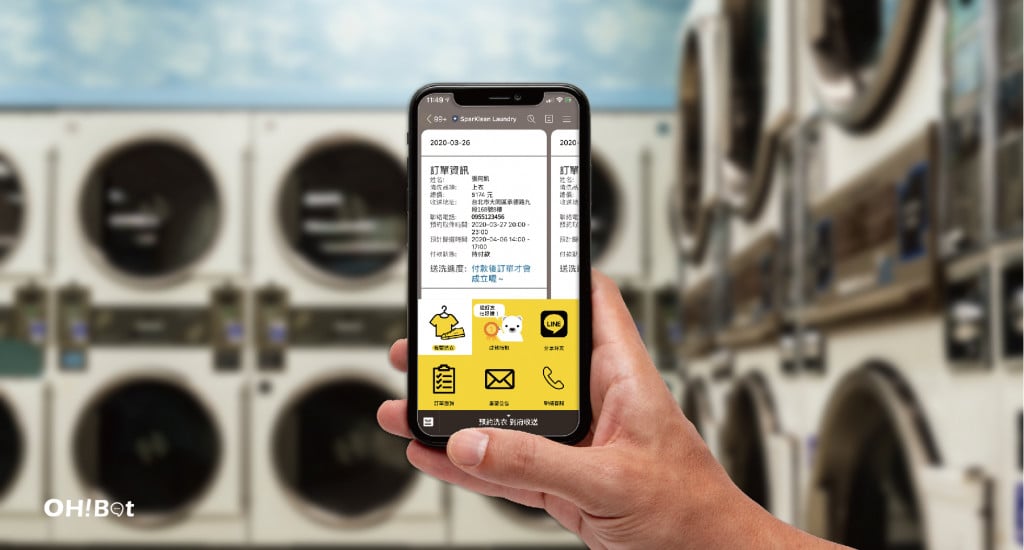
▲At-Home Laundry Smart Service System
Lowering the barrier to using the service, effectively improving customer service satisfaction!
The dry cleaning brand chain store initially required downloading the APP for use; however, after implementing AI chat-bot technology, it has been converted to only requiring addition to LINE@ for use. The switch in service entry points has already significantly boosted consumer willingness to use during the pilot phase, with corresponding increases in orders and sales.
Future expansions will include online keyword advertising as well as in-store promotions, and a marketing strategy 'Old members invite new friends for discounts' has been planned. The system is also applied to the food and beverage industry, and will continue to be promoted to other suitable industries.
The dry cleaning brand chain store has planned to establish 'small outlets', reducing the personnel needed to check orders and clothes, and has contacted locker services for collaborations to serve customers more broadly.
「Translated content is generated by ChatGPT and is for reference only. Translation date:2024-05-19」