【2021 Application Example】 Fongyu Uses AI Knowledge-based Fish Farming to Effectively Increase Aquatic Production by 10%

Fisheries is an important industry in an island economy. However, the fish farming industry has faced severe challenges in recent years, including climate change, labor shortage, and rising costs. In particular, nearly 110,000 workers in agriculture will retire due to old age over the next 10 years. For this reason, the need for aquaculture to move towards smart farming is becoming increasingly urgent.
Founded in 2014, Fongyu Corp. Ltd. has developed a unique eco-friendly farming model based on its own fish farming. It uses AI knowledge-based fish farming to effectively increase aquatic product production by 10%, and reduced labor cost by 15%.
The word "Fongyu" has a profound meaning. "Fong" represents good mountains and "Yu" represents good water, and is the hope that companies will allow Taiwan to always have good mountains and good water.It is also a homophone for "having a full figure," expressing the hope that products will give consumers a full and healthy body and mind. The founder of the company, Liu Chien-Shen, has been through the difficult entrepreneurial journey of becoming an apprentice in fish farming, raising funds, renting fish farms, establishing a fish farming company, building a brand, and expanding sales.
Labor shortage and aging workers are hidden worries in the fish farming industry
Currently, fish farms in Taiwan are still mainly traditional fish farms, and farming techniques are still passed down through word-of-mouth. In addition, the labor shortage and average age of workers exceeding 60 years old has made it impossible to effectively stably improve productivity and yield. This farming method makes it difficult to prevent and control diseases, and greatly increases the possibility of excessive use of drugs, environmental pollution, and water quality and ecological damage, creating a vicious cycle that lowers the quality of fish farming.
In addition, 65.1% of workers in Taiwan's fish farming industry are inadequately skilled. With limited support from IoT sensors, traditional fish farmers still mainly rely on their own experience and knowledge for water quality management, feeding, and disease detection. Fish farming management relies heavily on the ability of individual fishermen. Once experienced workers retire, the industry will not only face the issue of succession, but also the difficult of stably supplying a certain amount of harvest that meets quality standards. This may cause a dilemma for the entire industry from fish farming to sales.
In order to improve the pain point of inability to pass on experience in fish farming, and at the same time create a "digital" foundation for fish farming, the top priority must be to collect farming behavior data and develop AI services as an important starting point.
Fishery digital twin technology helps fishermen transition to smart farming
With the assistance of the Institute for Information Technology (III), Fongyu implemented the "fishery digital twin" technology to dynamically adjust the farming schedule. In other words, the fish farming schedule is adjusted according to the species, habits, and variables of the fish. The use of AI in fish farming not only effectively increase aquatic production by 10%, but also reduced labor cost by 15%.
In terms of specific methods, we first digitalized the fish ponds, feed, and decision-making behavior for each species, such as sea bass and Taiwan tilapia, and recorded the seasonal temperature changes from releasing seedlings to harvesting, all of which were digitalized, gradually recording the experience and methods of experienced workers into a rich database. Based on the recorded data, we analyzed the compound variables to find the best farming behavior and generate a dynamic farming schedule.
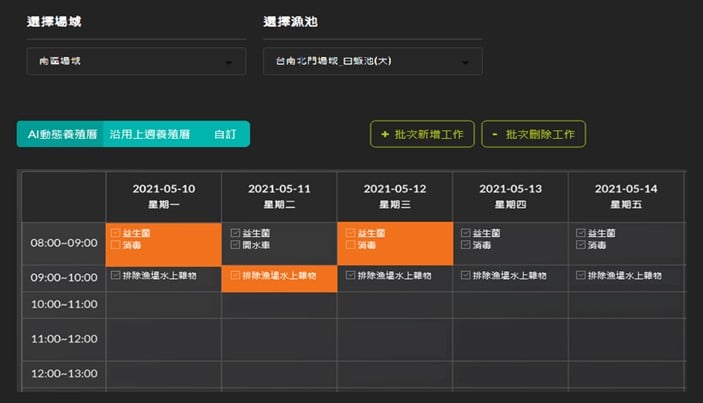
▲ The records for each pool provide data on workers' experience.
However, fish farming behavior generally relies on rules of thumb. Even experienced fish farmers cannot ensure that they will find the best solution. Therefore, new methods are proposed to solve this issue: That is, "to determine the best fish farming behavior by predicting the interaction with water quality and past data on feeding, and evaluating fish farming behavior based on water quality and fish farming," and provide fishermen with the most intuitive recommendations through daily schedules. To continue optimizing the dynamic fish farming calendar on a rolling basis, iterations of the model will be developed through the three-step cycle:
(1) Input the current fish farming calendar into the model;
(2) The model predicts the future environment;
(3) Shortcomings of the fish farming calendar are corrected based on the future environment to obtain a new version of the fish farming calendar.
In the process, the experience of aquaculture experts is used to establish the causal relationship between fish farming behavior and the environment. The establishment of a dynamic fish farming process and technology-based fish farming recommendation services provide a traceable and detailed fish farming process. It is one of the few technologies that can digitalize fish farming. Fishermen can quickly and easily record their daily behaviors to build knowledge without taking up too much time, but in the long run it can reduce labor cost by 15% and increase output and revenue by an average of 10%.
Smart fish farming has achieved outstanding results, reducing labor cost by 15% and increasing output by 10%
At the same time, the fish farming calendar can also be extended to different aquatic species, such as white shrimp, milkfish, clams, and Taiwan tilapia, to produce fish farming schedules for ponds with different specifications, and the harvested aquatic species can be traced according to different specifications, establishing vertically integrated services for safe food products. Fongyu's main products are divided into two categories. One is aquaculture modules, including fry, feed, materials and probiotics, production planning and processes, and monitoring, which can be sold separately or exported as modules.
▲The high-quality aquatic products produced by Fongyu have repeatedly won awards. (Figure: Fongyu’s official website)
The other category is high-quality aquatic products, including seabass fillets, seabass balls, oil-free seabass balls, seabass dumplings, and seabass soup. The products have won various awards, including the top ten souvenirs in Pingtung in 2017, "Barramundi Fillet" won the 2017 Eatender of the Council of Agriculture (COA), "Oil-Free Barramundi Fillet" won the 2018 Eatender Gold Food Award of the COA, and "Dumplings of Barramundi" and "Barramundi Broth" won the 2019 Eatender of the COA. The consecutive awards represent that the "quality" of Fongyu’s aquatic products can be seen and eaten with peace of mind.
In addition, Fongyu has exclusive fingerlings that meet international needs, such as: Pure seawater cultured tilapia fingerlings and seawater Taiwan tilapia fingerlings from selective breeding (FY-01) are items that aquaculture companies in many countries are looking forward to. The company also has aquaculture modules, disease monitoring tools, and feeding materials designed in accordance with the environment, in order to provide customers with more stable income.